Unlocking Opportunities: The Role of Healthcare Datasets for Machine Learning
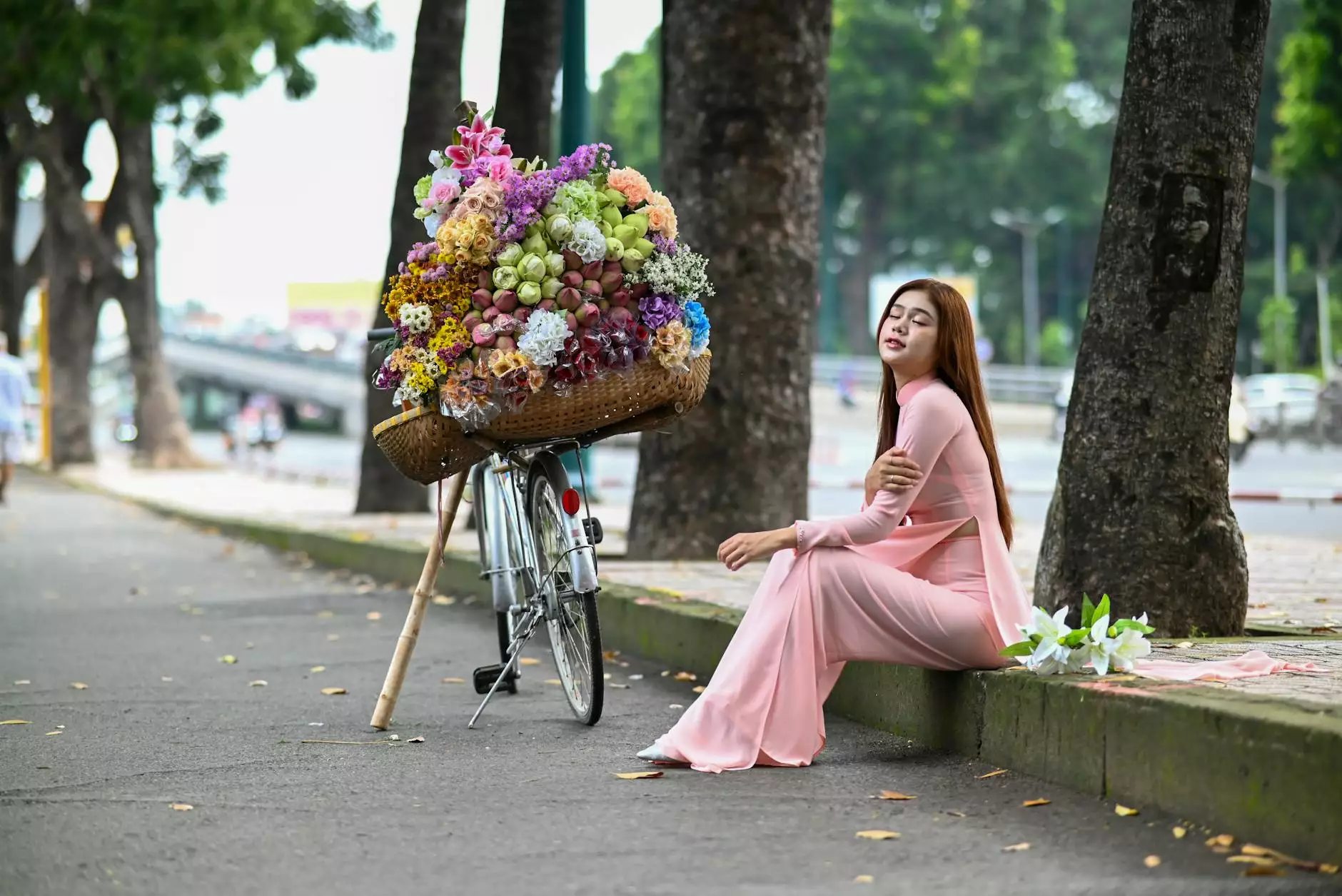
In an era characterized by rapid technological advancements, the utilization of healthcare datasets for machine learning is gaining traction as a pivotal element in the transformation of the healthcare industry. The intersection of artificial intelligence (AI) and healthcare presents a myriad of opportunities that not only enhance patient care but also streamline operational efficiency within healthcare organizations. This article delves deep into the significance, applications, and future implications of healthcare datasets in the realm of machine learning.
Understanding Healthcare Datasets
Healthcare datasets consist of various forms of data collected from healthcare environments, including clinical records, patient demographics, treatment protocols, and more. These datasets serve as a backbone for machine learning applications, providing the necessary information for algorithms to learn from and make predictions.
Types of Healthcare Datasets
- Electronic Health Records (EHRs): Comprehensive digital versions of patients' paper charts that provide real-time, patient-centered records.
- Clinical Trials Data: Data derived from clinical trials, including patient outcomes and treatment efficacy.
- Genomic Data: Patient genetic information that aids in personalized medicine.
- Operational Data: Information about the efficiency of services provided by healthcare institutions.
- Patient Surveys and Feedback: Data obtained from patient experiences that assess satisfaction and identify areas for improvement.
The Importance of Data Quality
For machine learning models to deliver reliable results, the quality of healthcare datasets for machine learning cannot be overstated. High-quality data ensures that the predictive models are accurate and applicable to real-world scenarios. In healthcare, poor data quality can lead to erroneous conclusions, affecting treatment decisions and long-term patient outcomes.
To maintain data quality, healthcare organizations must prioritize:
- Data Integrity: Ensuring the accuracy and consistency of data across records.
- Completeness: Data should be exhaustive, capturing all relevant patient information.
- Timeliness: Data must be up-to-date to be relevant for current practices.
Applications of Machine Learning in Healthcare
The applications of machine learning powered by healthcare datasets are vast and varied. Below are some key areas where machine learning has made significant strides:
Predictive Analytics
Predictive analytics involves using statistical algorithms and machine learning techniques to identify the likelihood of future outcomes based on historical data. In healthcare, this can mean predicting patient readmission rates, assessing the risk of developing chronic diseases, or forecasting sudden health events. Such insights allow healthcare providers to implement proactive interventions, enhancing patient care.
Personalized Medicine
Machine learning enables the analysis of genomic and clinical data to tailor treatment plans based on individual patient profiles. By considering a patient’s unique genetic makeup, healthcare professionals can determine the most effective therapies, significantly improving treatment outcomes.
Treatment Optimization
Effective treatment plans can be formulated by analyzing past medical data. By understanding how different patients responded to various treatments, healthcare providers can refine their approaches, ensuring better efficacy and reduced side effects for patients.
Drug Discovery
The process of developing new medications is often lengthy and expensive. Machine learning accelerates drug discovery by analyzing vast datasets encompassing chemical compounds, biological activity, and patient outcomes. This results in faster identification of promising candidates for clinical trials.
Challenges in Utilizing Healthcare Datasets
While the potential of healthcare datasets for machine learning is vast, there are inherent challenges that organizations must navigate:
Data Privacy and Security
Patient data is sensitively protected under regulations such as HIPAA (Health Insurance Portability and Accountability Act). Ensuring the security and privacy of healthcare datasets is crucial while leveraging them for machine learning. Organizations must implement robust cybersecurity measures and comply with legal standards.
Interoperability Issues
Many healthcare systems use disparate electronic health record systems that lack standardization. The challenge lies in integrating these systems to create cohesive datasets for analysis. Ensuring data interoperability facilitates smoother machine learning implementations by providing comprehensive datasets.
Skills Gap
The successful application of machine learning in healthcare requires a blend of domain expertise and data science skills. Bridging the skills gap through education and training is essential for healthcare professionals to harness the power of data effectively.
Future Trends in Healthcare Machine Learning
As technology continues to evolve, the future of healthcare datasets and machine learning looks promising. Here are some trends to anticipate:
Real-Time Data Analysis
With the increasing adoption of wearable technology and IoT devices, real-time data collection and analysis will become more common. This will enable healthcare providers to make immediate decisions based on the most current patient data, improving care outcomes significantly.
Blockchain for Data Security
Blockchain technology holds potential for enhancing data security and interoperability. By creating a decentralized and secure method of storing patient information, healthcare organizations can ensure data integrity and privacy.
Enhanced Predictive Modeling
As machine learning algorithms improve, predictive models will become more sophisticated, allowing for more accurate forecasts and personalized patient interventions.
Conclusion
The integration of healthcare datasets for machine learning is undeniably revolutionizing the healthcare landscape. From predictive analytics to personalized medicine, the implications for patient care and operational efficiency are profound. However, with these opportunities come challenges that require attention, particularly regarding data quality, interoperability, and privacy.
As we move forward, stakeholders in the healthcare sector must coalesce around best practices for data management while investing in technology and human capital to fully realize the potential of machine learning. The future is bright for healthcare powered by intelligent data solutions—an exciting frontier awaits those willing to embrace it.