The Importance of **Bounding Boxes** in Data Annotation Tools
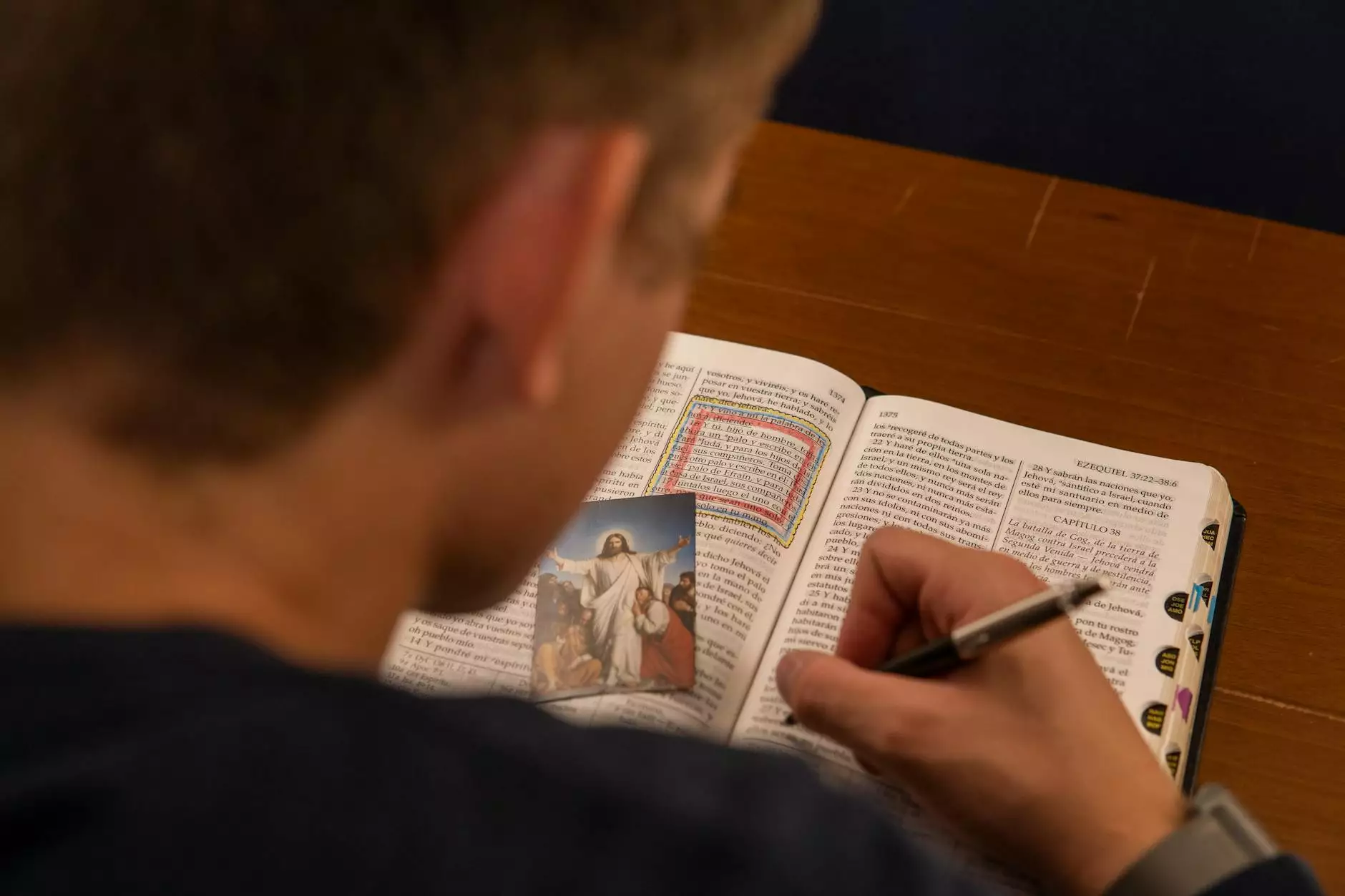
In the fast-evolving world of artificial intelligence and machine learning, one term that is crucial yet often overlooked is the bounding box. As businesses increasingly rely on data-driven decisions, the role of effective data annotation tools becomes paramount. This article delves into the significance of bounding boxes in data annotation, exploring how they enhance AI training models, facilitate computer vision applications, and contribute to better business outcomes.
Understanding the Bounding Box Concept
A bounding box is often defined as a rectangular box that encapsulates an object within an image. This concept plays a vital role in various applications, especially in fields like computer vision and image processing. By framing the object of interest, bounding boxes provide essential metadata that helps algorithms learn to identify and categorize objects. In data annotation tasks, they serve as the primary signal to machines, guiding them in recognizing patterns and making determinations based on visual data.
Why Are Bounding Boxes Essential in Data Annotation?
The effectiveness of AI models significantly hinges on the quality of data fed into them. Here are key reasons that underscore the importance of bounding boxes in data annotation:
- Object Localization: Bounding boxes allow for precise localization of objects within images. This task is crucial in fields such as autonomous driving, where a vehicle must identify pedestrians, other vehicles, and obstacles.
- Enhanced Model Training: Using bounding boxes in annotated images enables models to learn which features correspond to which objects, thereby enhancing their accuracy during inference.
- Data Segmentation: Bounding boxes assist in differentiating various objects within the same image, enhancing the granularity of data segmentation processes.
- Improved Annotation Efficiency: Implementing bounding boxes in annotation tools allows for quicker and more efficient marking of various objects, accelerating the overall training process.
- Higher Quality Data: By using structured annotation methods like bounding boxes, companies can establish a standardized data quality framework, which is vital for AI reliability.
The Role of Bounding Boxes in Computer Vision
In computer vision, the bounding box acts as a landmark that enables machines to understand the spatial relationships between different objects in a video or image. In particular, the importance of bounding boxes can be observed in several domains:
1. Autonomous Vehicles
For autonomous vehicles, understanding their environment is key to ensuring safety. Bounding boxes are used to categorize and localize different entities (vehicles, pedestrians, traffic lights) in real-time. With this information, the vehicle’s AI can make informed decisions, such as when to stop, accelerate, or turn, thereby reducing the chances of accidents.
2. Facial Recognition
In facial recognition systems, bounding boxes help delineate faces from the background, enabling algorithms to focus on facial features. This process is crucial for security systems, social media tagging features, and even customer behavior analytics in retail environments.
3. Retail and Inventory Management
Retailers utilize bounding boxes to automate inventory management. By annotating product images with bounding boxes, AI systems can accurately track stock levels, identify restocking needs, and reduce inventory discrepancies.
Implementing Bounding Boxes in Data Annotation Tools
For businesses looking to incorporate a robust data annotation strategy, understanding how to effectively implement bounding boxes is critical. Below are some practical strategies that organizations can employ.
1. Choose the Right Annotation Tool
The first step to effective data annotation is selecting a tool that supports the creation of bounding boxes. Platforms like Keylabs.ai offer user-friendly interfaces and advanced features tailored for optimal object recognition missions. Look for tools that provide:
- Intuitive user interfaces for easier bounding box creation.
- Collaboration features for teams to work on large datasets.
- Integration capabilities with machine learning frameworks.
- Quality control mechanisms to ensure accuracy in annotation.
2. Train Your Team
Having a tool is only part of the equation; training your team to use these tools effectively is equally essential. Provide comprehensive training that covers:
- The importance of accuracy in bounding box placement.
- How to handle complex images with multiple overlapping objects.
- Best practices for managing ambiguity in visual data.
3. Regularly Review Annotations
Implement periodic review sessions to assess the quality of annotations. This process includes checking whether bounding boxes are correctly placed and conform to the guidelines. Feedback loops are critical in refining the annotation process and ensuring continuous improvement.
Case Studies: Success Stories Using Bounding Boxes
Numerous companies across various sectors have successfully integrated bounding boxes in their data annotation processes. Here are a couple of noteworthy examples:
1. Tech Giants and AI Development
Companies like Google and Facebook leverage bounding boxes in their image recognition systems. These tech giants have developed vast datasets annotated with bounding boxes, which enable their AI systems to accurately categorize and identify millions of images daily.
2. Healthcare Innovations
In the healthcare industry, startups focusing on medical imaging have employed bounding boxes to train models that assist in diagnosing conditions through imaging scans. By accurately annotating medical images, these companies facilitate the development of advanced diagnostic tools that improve patient outcomes.
The Future of Bounding Boxes in AI and Data Annotation
As AI continues to evolve, so too will the methods and technologies associated with data annotation. The future of bounding boxes will likely be shaped by advancements in several areas:
- Automated Annotation: With the rise of deep learning, future tools may automate the creation of bounding boxes, decreasing the reliance on manual processes.
- 3D Bounding Boxes: As 3D environments become more relevant (e.g., in AR and VR applications), the need for three-dimensional bounding box annotation will grow, offering richer data for training models.
- Integration with Other Data Types: Future data annotation platforms may integrate bounding boxes with other forms of data, such as text and audio, allowing for a more comprehensive understanding of multimedia data.
Conclusion
The concept of the bounding box is not merely a technical detail; it is at the heart of data annotation for machine learning and AI applications. As organizations continue to appreciate the value of high-quality annotated data, understanding and implementing effective strategies for bounding box annotation will only become more critical. Through thoughtful application of these principles and tools, businesses stand to gain a significant competitive advantage, ensuring they harness the full potential of AI and data analytics.
Keylabs.ai excels in providing a cutting-edge Data Annotation Tool and Data Annotation Platform that includes robust binding box functionalities, ensuring organizations can streamline their data annotation efforts while maintaining high quality. Embracing these solutions will not only enhance operational efficiency but also accelerate the journey toward AI-driven innovation.