Transforming Businesses with Data Labelling Services
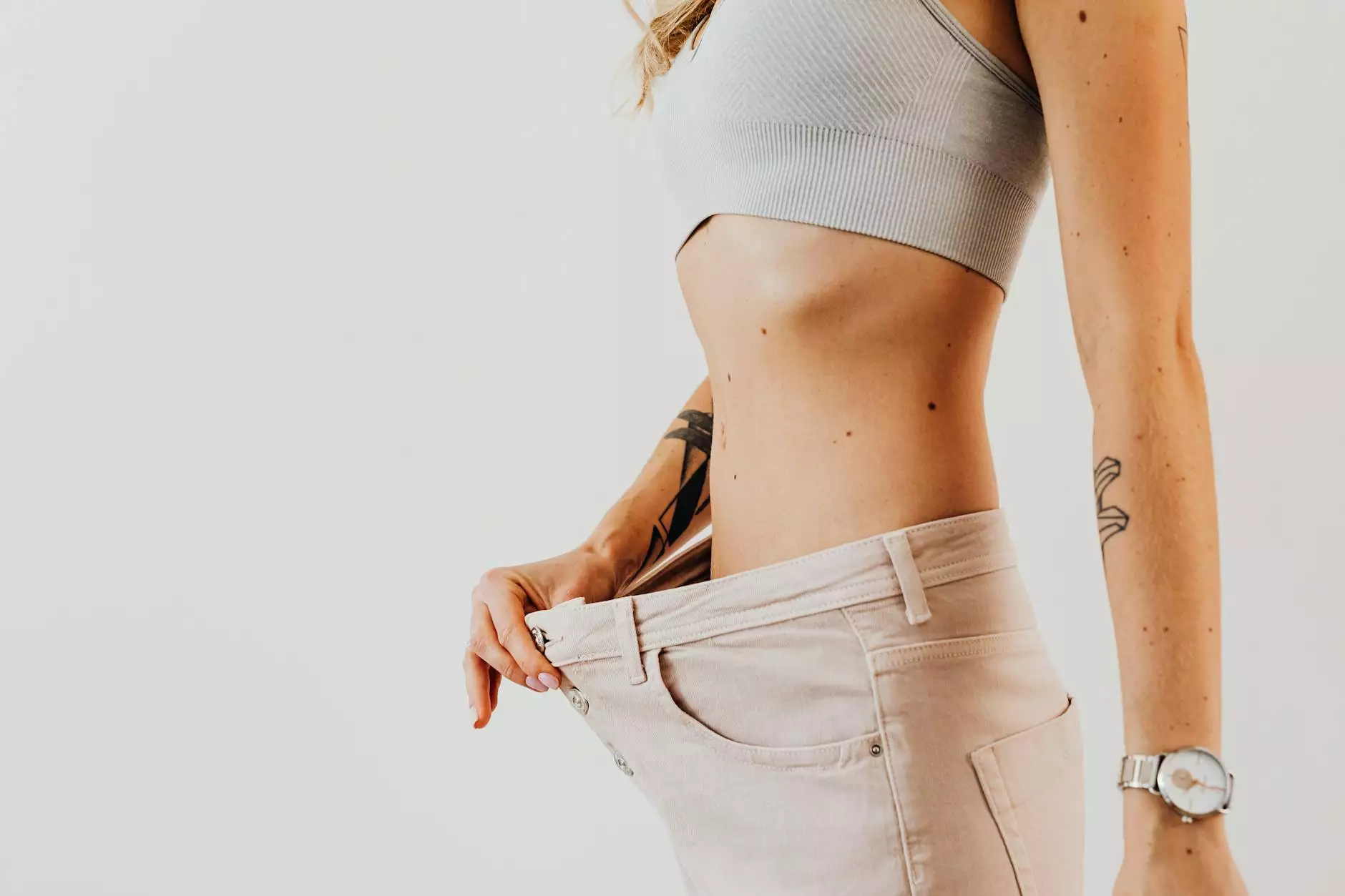
In today’s fast-paced digital landscape, the integration of artificial intelligence (AI) and machine learning (ML) into business strategies has become more critical than ever. A cornerstone of these technologies lies in data labelling services, which empower organizations to enhance their AI models and streamline their operations. This article delves deep into the world of data labelling, exploring its significance, uses, and how it can reshape your business.
What is Data Labelling?
Data labelling is the process of tagging or annotating data to make it understandable for machine learning models. The labelled data serves as a foundation for training AI algorithms, enabling them to learn from human input. Without accurate data labelling, businesses may struggle to deploy effective AI solutions, ultimately hindering their innovation and growth.
Importance of Data Labelling Services for Businesses
As businesses increasingly rely on data-driven decisions, the role of data labelling services becomes paramount. Here’s why:
- Enhances Model Accuracy: Well-labelled data is crucial for teaching models to recognize patterns accurately. Quality annotations lead to better predictions and insights.
- Supports Diverse Applications: From image and video recognition to natural language processing, data labelling services bridge the gap between raw data and actionable intelligence.
- Facilitates Compliance: In regulated industries, having accurately labelled data can help organizations stay compliant with legal standards and regulations.
- Enables Scalability: As businesses grow, so does the volume of data they handle. Reliable data labelling services can scale to meet these increasing demands.
Types of Data Labelling Services
Data labelling encompasses various techniques tailored to different types of data. Understanding these methods can help businesses select the right service for their needs:
1. Image and Video Annotation
In applications such as autonomous driving and facial recognition, image and video annotation are critical. This includes:
- Bounding Box Annotation: Drawing boxes around objects within images.
- Semantic Segmentation: Classifying each pixel in an image to enhance object recognition.
- Object Tracking: Following objects across frames in a video.
2. Text Annotation
In the realm of natural language processing, text annotation is vital. Common practices include:
- Sentiment Analysis: Tagging text to determine sentiment, whether positive, negative, or neutral.
- Named Entity Recognition (NER): Identifying and categorizing entities in text such as names and locations.
- Intent Recognition: Understanding user intentions in chatbots and virtual assistants.
3. Audio Annotation
For voice recognition and audio processing, data labelling services include:
- Speech Recognition: Transcribing spoken language into text.
- Emotion Detection: Tagging audio files to convey the emotional tone of speech.
Choosing the Right Data Annotation Platform
With the rise in demand for data labelling services, businesses must choose platforms that align with their objectives. Here are key considerations:
- Quality Assurance: The platform should ensure high standards in data quality through regular audits and validations.
- Scalability: The ability to handle large datasets efficiently is essential.
- Flexibility: A good annotation platform offers a variety of labelling tools to suit different projects.
- Integration Capabilities: The platform should allow easy integration with existing systems.
Benefits of Collaborating with Professional Data Labelling Services
Opting for professional data annotators can deliver numerous advantages to businesses:
1. Expertise and Experience
Professional service providers are equipped with the necessary expertise to deliver high-quality annotations efficiently. Their experience ensures that they can handle complex projects that may challenge in-house teams.
2. Cost-Effectiveness
While it might seem advantageous to manage labelling in-house, outsourcing can often reduce costs by saving time and resources. Businesses can focus on their core activities while outsourcing their data labelling needs.
3. Time Efficiency
Fast-paced markets demand quick turnarounds. Leveraging third-party services can enhance productivity by delivering timely results without compromising quality.
Impact of Data Labelling on Machine Learning Projects
In machine learning, the quality of data influences the effectiveness of a model significantly. Here’s how data labelling directly impacts projects:
1. Enhanced Predictive Power
With quality labelled data, machine learning models can learn more accurately which translates to improved predictive capabilities. This is crucial for decision-making processes.
2. Reduced Bias and Improved Fairness
Proper data labelling can help in identifying and mitigating biases in datasets, leading to fairer outcomes in AI applications.
3. Better ROI on AI Initiatives
Investing in precise data annotation ensures that businesses maximize their return on investment on AI projects. Well-trained models yield better results, leading to increased proficiency in operations.
Future Trends in Data Labelling Services
The data labelling landscape is evolving rapidly. Here are some anticipated trends:
- Automated Data Labelling: Technological advancements may see a rise in automated labelling, where algorithms assist in the initial labelling process.
- Crowdsourced Annotations: Crowdsourcing can enable vast, diverse datasets that cater to multiple perspectives, improving model training.
- Precision Data Labelling: The need for niche labelling professionals will rise, as industries will require specialized knowledge for accurate data annotation.
Conclusion
In conclusion, data labelling services play a vital role in ensuring businesses leverage the power of AI and machine learning effectively. By investing in quality annotation platforms like keylabs.ai, companies can enhance their data-driven strategies, improve model accuracy, and ultimately achieve their business goals. As the industry continues to evolve, staying informed about best practices in data labelling will be crucial for any organization looking to harness the full potential of their data.